8 data analytics challenges—and smart solutions to solve them
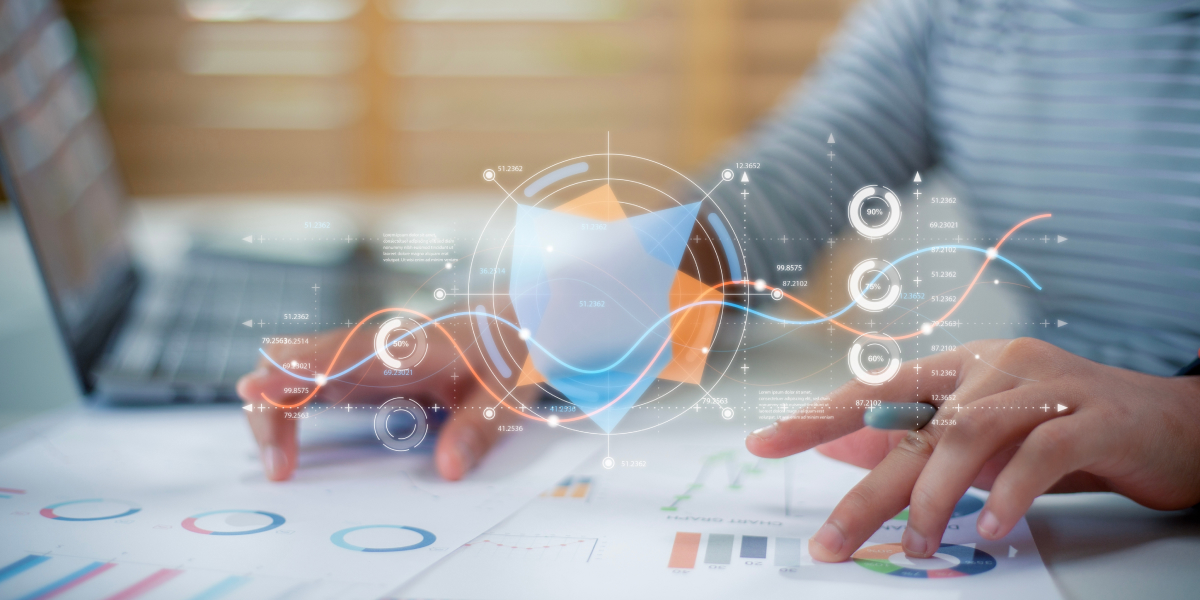
Highlights
- The global data analytics market is projected to reach $190 billion by 2028, yet many businesses struggle to extract real value from their data due to common challenges.
- Data quality issues, integration with legacy systems, and scalability bottlenecks are among the top hurdles preventing organizations from fully leveraging analytics.
- Talent shortages and misalignment with business goals hinder effective implementation, limiting organizations from making data-driven decisions.
- Ethical concerns, data privacy regulations, and operational roadblocks create additional complexities, making it harder for businesses to balance compliance with effective analytics.
- AI-powered data governance, hybrid integration, and scalable cloud solutions help businesses overcome these challenges by improving data quality, connectivity, and performance.
By 2028, the global data analytics market is expected to reach $190 billion. In anticipation, businesses are tapping into analytics-driven insights to make smarter decisions, boost efficiency, and control risks. However, certain data analytics challenges prevent organizations from fully capitalizing on its benefits.
Through research, Tamr, Inc. a leading cloud native data mastering solution estimated that more than 500 data leaders had data initiatives marked for 2023. However, half of these aspirants continued to struggle realizing business value from their company data.
This blog unpacks such hurdles and provides actionable solutions, helping you turn obstacles into opportunities.
8 data analytics challenges
Below are 8 common data analytics challenges and actionable solutions.
Data quality issues
Inconsistent, incomplete, or duplicate data leads to inaccurate insights and decision-making errors. Furthermore, data silos amplify quality issues, making it one of the most critical data analytics challenges.
Integration with legacy systems
Legacy systems often fail at handling modern analytics workloads, creating bottlenecks in integrating new technologies or datasets. These outdated infrastructures lack the scalability, processing power, and flexibility required for advanced analytics, slowing down data processing and limiting real-time insights.
Scalability and performance bottlenecks
As data volumes grow exponentially, traditional systems struggle to scale, leading to slow analytics and missed insights. Legacy architectures often lack the processing power and storage capacity needed to handle vast and complex datasets, resulting in delayed decision-making and inefficiencies.
Read more: Data observability: The key to reliable data pipelines
Talent shortages
A lack of skilled professionals with expertise fosters data analytics challenges, making it difficult to extract meaningful insights. Without the right talent, organizations struggle to clean, interpret, and apply data effectively, leading to inconsistent analyses and missed opportunities.
Misalignment with business goals
Analytics efforts often fail when they don’t align with organizational objectives, leading to irrelevant or unactionable insights. Besides, without a clear connection to business goals, data-driven initiatives can become siloed, wasting resources and failing to drive meaningful impact.
Ethical and bias concerns
Bias in data collection and modeling can lead to flawed or discriminatory outcomes, eroding trust in analytics. When data sets lack diversity or reflect historical prejudices, AI models can reinforce and amplify these biases, leading to inaccurate insights and unfair decision-making.
Balancing privacy with analytics
As data privacy regulations like GDPR and HIPAA tighten, businesses face challenges in maintaining compliance while using data effectively. Subsequently, stricter requirements on data collection, storage, and processing demand robust security measures, transparent policies, and continuous monitoring to avoid hefty fines and reputational damage.
Operationalizing insights
Many organizations struggle to turn analytical insights into real-world actions due to a disconnect between analytics teams and decision-makers. Data analytics challenges often stem from siloed operations and communication gaps.
8 technology-powered solutions for better outcomes
Here’s how each of the above 8 challenges can be fixed:
Better data quality
Robust data governance frameworks can help standardize data collection and management processes. In addition to that, clear data ownership policies and regular audits further enhance data integrity, reducing inconsistencies and redundancies. AI-driven data cleaning tools can automate error detection and correction, minimizing manual intervention and improving efficiency in handling large datasets, and overcoming data analytics challenges.
Hybrid integration
Hybrid integration solutions can connect legacy systems with modern cloud platforms, enabling seamless data exchange without requiring a full system overhaul. By leveraging middleware and integration platforms as a service (iPaaS), businesses can create a unified data environment that supports real-time analytics.
Enhanced scalability and performance
Transitioning to distributed computing frameworks like Hadoop or Apache Spark allows businesses to process vast amounts of data in parallel, improving speed and efficiency. Moreover, implementing auto-scaling cloud solutions ensures that infrastructure adapts dynamically to workload demands, reducing bottlenecks and improving performance.
Employee training
Upskilling existing employees with targeted training programs in analytics tools and methods ensures that teams stay ahead of evolving data trends, mitigating data analytics challenges like talent shortages. Besides, hands-on workshops and certifications in data visualization, statistical analysis, and AI/ML applications can boost analytical capabilities across the organization.
Read more: Dancing with the data: AI tools that empower you to make decisions with finesse
Data-driven culture
Involving key stakeholders in defining analytics objectives ensures alignment with business priorities and maximizes the impact of data-driven initiatives. Establishing clear KPIs and performance metrics tied to analytics efforts helps track progress and demonstrate ROI.
Ethical guidelines
Using diverse datasets can mitigate bias in machine learning models, ensuring fairer and more representative outcomes across different demographics. Regular bias audits and fairness assessments can help identify and rectify unintended discrimination in AI-driven analytics.
Synthetic data
Employing synthetic data allows organizations to perform analytics and train machine learning models without compromising sensitive information, enhancing data privacy and mitigating data analytics challenges. This approach is particularly beneficial in industries like healthcare and finance, where regulatory compliance is stringent. Audit analytics processes regularly to ensure adherence to privacy standards and verify the accuracy of synthetic data in replicating real-world scenarios.
Cross-functional collaboration
Analytics platforms provide user-friendly dashboards and visualizations tailored to business leaders, making data insights more accessible and actionable. Moreover, embedding natural language processing (NLP) capabilities in analytics tools can allow non-technical users to interact with data intuitively. Cross-functional collaboration can bridge the gap between data scientists and business units, ensuring that insights are contextualized and aligned with strategic goals
Read more: Overcoming talent shortages in technology
To sum it up…
Addressing data analytics challenges is critical for organizations aiming to remain competitive in this data-driven business environment. Challenges like data silos, inconsistent quality, and integration with legacy systems can hinder decision-making, leading to missed opportunities and inefficiencies. As businesses increasingly rely on analytics to drive strategic decisions, failing to address these issues can impact operational efficiency and limit the ability to respond to market changes, customer needs, and emerging trends.
Modern tools and technologies like AI, GenAI, and data engineering frameworks play a pivotal role in solving these challenges by enabling automation, scalability, and enhanced decision-making capabilities. Additionally, these tools help organizations process large volumes of data in real-time, integrate disparate datasets seamlessly, and deliver insights through user-friendly platforms accessible to both technical and non-technical teams. Furthermore, by adopting advanced analytics solutions, businesses can unlock the true potential of their data, ensuring that every decision is informed, efficient, and forward-looking.
Ready to realize business value? Explore our data analytics solutions today.