AI automation: the business agility and scale enabler
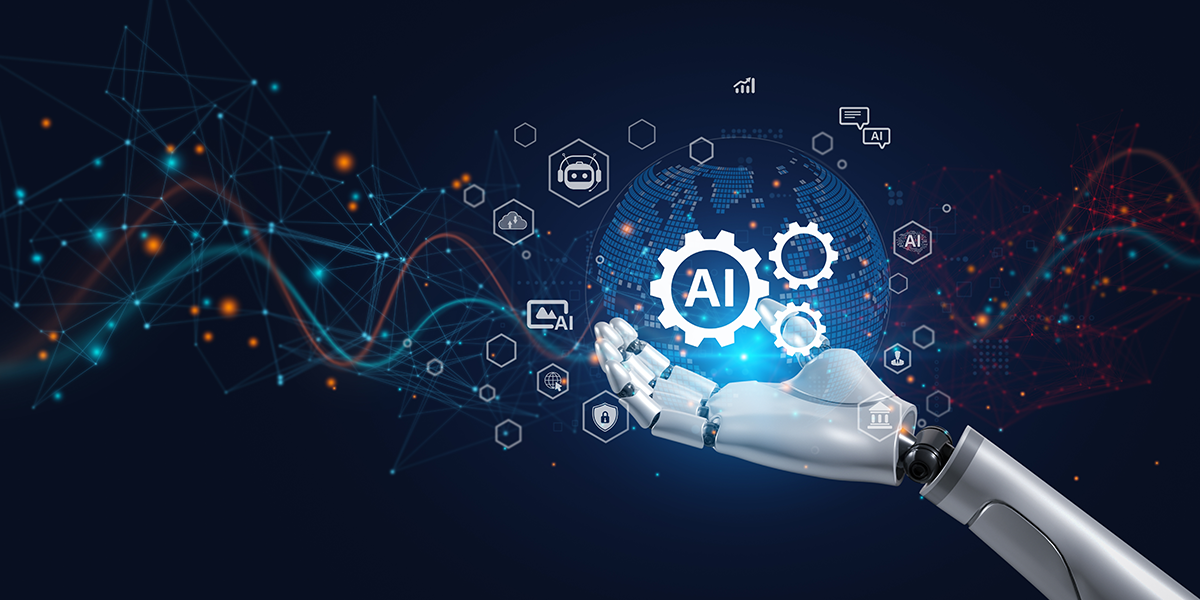
Highlights
- AI automation goes beyond rules-based workflows, introducing systems that learn, adapt, and forecast in real time—transforming how businesses operate and scale.
- Common pitfalls—like unclear goals, siloed systems, and employee resistance—can stall progress, but a strategy-led, people-first approach ensures adoption and impact.
- From predictive maintenance in manufacturing to fraud detection in finance, real-world examples show how AI automation is already delivering measurable outcomes.
- The future of automation is intelligent and collaborative, driven by self-learning models, generative AI, edge computing, and real-time decision-making.
From static systems to smart automation
AI automation is reshaping industries beyond recognition. In contrast to conventional automation, which is based on fixed rule-based systems and pre-programmed logic, AI-based automation introduces flexibility, learning, and forecasting into the mix. While legacy systems can only execute predetermined workflows, AI systems can learn from data continuously, adjust to evolving environments, and make decisions in real time.
For CTOs, COOs, and leaders of automation, the problem isn’t merely about applying AI. It’s about infusing it seamlessly, growing it effectively, and getting it to produce visible impact. Many organizations start out well but eventually encounter issues such as workforce resistance, data integration issues, unclear business objectives, or scalability difficulties.
This blog examines the development of AI automation, recognizes sectors that are leading the charge, outlines a step-by-step approach to adoption, and looks ahead at future possibilities. Upon completing this guide, you can expect to have all the information you need to make the AI automation leap for your business.
Where businesses go wrong
Despite its potential, the effective use of AI automation continues to be a major challenge for most organizations. Although the technology is obviously beneficial in terms of efficiency and scalability, organizations too frequently fail to achieve its full potential. Usual pitfalls—everything from poorly defined goals to bad data quality can make even the best-looking initiatives fall apart.
1. Automating without strategy
Some firms jump into automation without determining what they are trying to accomplish. They invest in costly AI software hoping to see quick payoffs, then find that the technology is not suited to their particular requirements.
The solution: begin with definitive business goals.
Ask yourself the following questions:-
What are we trying to fix?
Begin by identifying the pain points in your business. Slow customer service response times? Supply chain operation inefficiencies? High staff turnover? Too many companies rush into AI in search of sweeping change without first identifying why. Being precise about the problem, whether it’s automating tedious work, increasing decision accuracy, or growing a service—guarantees that your AI project is mission-driven. The more defined the problem, the simpler it will be to define success.
Which processes can AI actually improve?
Target areas where data is plentiful, tasks are routine, and decisions follow predictable paths. For instance, AI can be naturally used to automate invoice processing, review customer feedback in bulk, or predict inventory requirements. An exhaustive audit of workflows will determine where AI creates value compared to where human monitoring is essential.
How does AI fit into our long-term business strategy?
Technology must never be working in a vacuum. It must be working for your larger objectives, whether that’s growth, innovation, operational effectiveness, or customer satisfaction. Ask: is this AI project getting us to where we need to be in 3, 5, or 10 years? For instance, if your strategic aim is to deliver more personalized customer experiences, investing in AI-driven recommendation engines or chatbots may be a good fit. If you’re concerned with sustainability, predictive maintenance or energy optimization software might be a more suitable choice. By linking AI initiatives to long-term strategy, you make sure they’re not only technically successful, but strategically effective.
2. Not preparing employees
The human element is often the biggest challenge. Employees may fear that AI will replace their jobs, leading to resistance and underutilization of the new technology.
The solution: adopt a people-first approach
Clearly explain that automation is designed to support and enhance current roles.
The communication here is key: automation to assist tasks done by humans, not replace them. By explicitly stating this purpose, organizations can reduce fear and resistance, and establish trust throughout teams.
Show how AI integrates with existing workflows to improve efficiency without taking over human tasks.
For instance, AI can be integrated into customer service platforms to provide responses to agents in real time, or into HR systems to automate candidate screening—not to make decisions, but to support human judgment. Providing concrete use cases and before-and-after examples can make it easier for employees to grasp how these tools simplify their everyday work and make it more efficient, without disempowering their roles or skills.
Provide comprehensive training and change management initiatives to ensure a smooth and confident transition.
This involves both technical training (how to operate the tools) and strategic direction (how the tools align with the overall picture). Concurrently, a well-designed change management plan, involving open communication, feedback mechanisms, and leadership buy-in can address anxiety, mitigate uncertainty, and create a culture of adaptation and innovation.
3. Treating AI as a standalone tool
AI automation is most effective when it exists within a larger system. Far too many organizations apply AI in some siloed fashion without considering whether it will work well with current workflows, software, or data sources.
The solution: integrate artificial intelligence into end-to-end business processes.
Ensure seamless integration with core business systems (ERP, CRM, etc.)
The effectiveness of AI relies heavily on its capability to connect with and communicate through the systems in which business-critical information resides. These systems include enterprise resource planning (ERP), customer relationship management (CRM), supply chain management (SCM). By being integrated into these systems, AI applications can provide real-time insights, simplify complex workflows, and offer actionable intelligence in the very moments and systems in which decisions are being made.
Dissolve internal silos to enable data to flow freely
One of the biggest impediments to successful AI adoption is disparate data. When departments or teams work separately, each with its own tools, processes, and data repositories—AI can see only partially and provide only partial insights. To realize true AI potential, companies need to put data unification at the forefront. That involves establishing a centralized or federated data platform that allows secure, real-time data sharing between departments.
Prioritize strategies that streamline multiple business processes at once:
Most AI applications begin small, addressing a single function or department, which is an excellent proof of concept. However, lasting value emerges when AI is leveraged to automate entire end-to-end processes cutting across various business functions. Order-to-cash, procure-to-pay, or hire-to-retire processes cut across various teams and systems, for instance. By examining the ways that AI automation can enhance end-to-end processes (instead of focusing on discrete functions) companies can realize compounding returns, enable cross-functional alignment, and achieve maximum return on investment.
Steering clear of these hazards is key to realizing the true potential of powering automation with AI. Equipped with an effective strategy and adequate preparation, businesses can leap over these recurring pitfalls and facilitate sustainable growth.
How to create an effective roadmap
While it may be tempting to implement AI automation all at once, businesses should remember that Rome wasn’t built in a day. They must integrate AI in a planned and phased manner. By developing a clear roadmap, companies can incorporate the technology seamlessly, scale it as they grow, and ensure it delivers long-term value.. Below is a step-by-step guide to help with your journey.
- Begin with a concise problem statement
Define the precise challenges your company is experiencing. Identify which processes are wasteful or error-prone, and where AI will have the greatest impact. Well-defined goals are necessary to avoid technology investments being misaligned with business requirements. - Ensure high-quality data
The cornerstone of AI is solid data. Clean, organized data is essential for building accurate models. Invest in sound data management habits that keep your information up-to-date, consistent, and reliable. Quality data will power superior insights and more trustworthy results. - Focus on employee adoption
At the center of any successful change are the people. Get your people involved early by demonstrating how AI can free them up and simplify routine work. Provide detailed training sessions and ongoing support to smooth the process. When staff get it and believe in it, resistance evaporates, and output increases. - Scale strategically
Begin small with testing on a selected region or function. Closely watch the results and elicit feedback from everybody involved in the AI implementation process. With success shown at a modest level, systematically introduce the solution throughout other domains in your firm. Phasing means reduced risk as well as continued process refining. - Continuously optimize
AI is not a project but a continuous journey. Set key performance indicators (KPIs) to measure success and areas where improvement is needed. Periodic reviews and incremental changes are required to keep up with evolving business requirements and market dynamics. Continuous optimization helps ensure that your strategy is effective and goal-oriented.
By following these steps, you can develop an end-to-end roadmap that optimizes operations and fuels strategic innovation.
Industries leading the way: a brief summary
In many industries, entrepreneurs are leveraging AI automation to reinvent operations and deliver exceptional customer experiences. These are some of the sectors that are setting the pace so you can get inspiration on building an effective roadmap:-
Manufacturing: How AI Is ending unexpected equipment failures
Manufacturers have long embraced automation, and AI is now taking their operations to even greater heights. Siemens, for example, has installed AI-driven predictive maintenance solutions in manufacturing settings to identify anomalies and predict equipment failure before it occurs. In a specific application, Siemens used machine learning algorithms to parse sensor data on production lines and allow for real-time insights and decreased unplanned downtime.
Today’s factories make use of AI-driven predictive maintenance to watch equipment around the clock. Through the analysis of thousands of sensor readings, these systems have the ability to predict machine failures before they occur. This preventative maintenance strategy slashes unplanned downtime, increases equipment longevity, and preserves otherwise lost productivity. Intelligent factories are not only more productive, they are changing the benchmarks for quality and process excellence.
Finance: detection of fraud in milliseconds
In 2023, HSBC introduced an AI-powered fraud detection system that can scan more than 650 million transactions per day, flagging suspicious activity in milliseconds and cutting false positives by more than 60%. This innovation illustrates how AI automation is fundamentally transforming fraud detection in finance.
Under conventional finance systems, fraud detection had been accomplished by static rule-based models that initiated alarms when pre-defined conditions had been met, like unusually high-value transactions or activity from suspicious locations. Although this approach could identify known patterns of fraud, it had difficulty with adaptability, tending to cause delayed detection and a large number of false positives. These systems had to be manually updated to account for new threats, and investigations tended to be reactive, occurring after suspicious transactions had already been made.
AI-driven fraud detection significantly enhances speed and precision by processing huge amounts of transactional data in real time. Rather than relying on fixed rules, AI models learn from past behavior and continuously evolve to detect subtle patterns in multiple dimensions— spending habits, device activity, and geolocation. This allows banks to detect and respond to suspected fraud in milliseconds, preventing threats from growing. The outcome is a system that operates proactively not only to curb fraud loss but also enhance customer confidence through limited disruption of rightful activity.
Retail: AI-powered forecasting Is reinventing retail inventory
Walmart deploys an AI-driven demand forecasting platform in its U.S. stores, allowing it to forecast changes in consumer buying behavior with unmatched precision. It decreased stockouts and overstocking while increasing product availability. The takeaway? The future of retail belongs to AI-driven supply chain optimization.
Retailers are also enjoying the benefits of artificial intelligence in automation, most notably in demand forecasting and inventory management.
Historically, retailers were dependent on historical point-of-sale information and seasonal patterns to predict demand and inventory. These static models rarely could factor in abrupt changes in shopper behavior, new market trends, or outside influences such as weather, promotions, or economic changes. Therefore, inventory planning was often miss-targeted, resulting in overstocking, stockouts, or wasteful warehousing expenses. Inventory level adjustments were reactive and time-consuming, and it caused supply chain delays and lost sales.
AI models can forecast what products will be in demand and when based on analyzing consumer behavior and market trends. This dynamic inventory management keeps the risk of stockouts or overstock at bay and reduces storage expenses. The outcome is a faster supply chain that reacts quickly to shifting markets, improving customer satisfaction and increasing the bottom line.
Read more: AI-powered demand forecasting transforming the future of retail
Healthcare: speeding up diagnostics and operations
In a landmark study, researchers at Stanford created an algorithm that could identify pneumonia from a chest X-ray more accurately than radiologists, reducing diagnostic time from hours to mere minutes. This type of innovation is how AI is increasingly changing healthcare in the modern world.
Historically, medical diagnostics have depended on laborious analysis of patient records and medical images by physicians and experts. Although successful, this method was time-consuming, prone to human error, and frequently hampered by the clinician’s capacity to spot subtle patterns—particularly in the early stages of diseases such as cancer. Administrative procedures like scheduling, data input, and record maintenance were also performed manually, taking up precious time that medical professionals could be spending with patients.
Clinics and hospitals are now using AI-powered diagnostic tools to scan patient data and medical images to enable the detection of diseases earlier and with more accuracy. For instance, AI has significantly increased the rates of early detection of cancer by picking up slight anomalies that are often missed by the human eye. Besides this, AI systems eradicate clerical tasks like patient scheduling and recordkeeping, allowing doctors to spend less time on these tasks and devote more time to patient care. Utilization of AI in all areas of healthcare is bringing enhanced performance and efficiency levels.
Logistics: route planning optimization
DHL is also already using AI to optimize last-mile delivery and routes in its worldwide logistics operations. The logistics brand leverages sophisticated machine learning algorithms to scan historical delivery patterns, real-time traffic flow, and weather conditions to optimize routes and make dynamic changes to delivery times. This enables drivers to bypass congestion, lower fuel costs, and increase delivery accuracy—particularly in high-demand seasons when efficiency is paramount.
Historically, logistics operations were based on fixed route planning and manual decision-making using pre-set delivery routes, past traffic habits, and set schedules. Such approaches were not real-time adjustable, and many times, deliveries were delayed because of unforeseen traffic, weather conditions, or vehicle malfunctions. Inefficiencies like pointless detours, downtime, and unnecessary fuel burn were prevalent, increasing operational expense and lowering overall reliability in delivery schedules.
With AI-powered systems monitoring real-time traffic, weather, and vehicle conditions, organizations can streamline routes, save fuel, and enhance delivery times. This adaptive strategy not only reduces delays but also saves operational costs by eliminating unnecessary mileage and fuel costs. Smart logistics networks are creating new standards for efficiency, reliability, and cost-effectiveness in supply chain management.
Throughout all these industries, the common thread is clear: AI automation is not just about speeding things up. It’s about making them smart. With AI in the mix, businesses can elevate their operating levels and become more competitive.
The future
The AI automation landscape is changing at lightning speed, and companies that invest in it now are setting themselves up for a competitive edge in the future. Some new trends are already setting the stage for the future:-
1. Self-learning AI models:
Upcoming automation systems will have self-learning abilities that enable them to get better with time without repeated human intervention. These models will learn from new data, and operations will become more efficient and reliable with time.
2. AI-driven cybersecurity automation:
As cyber threats become more sophisticated, AI automation is playing a key role in strengthening cybersecurity. By analyzing network traffic and user behavior in real time, these systems can detect vulnerabilities and prevent attacks before they occur. This proactive security measure is critical for protecting sensitive data and maintaining customer trust.
3. Automation powered by generative AI:
The fusion of generative AI with legacy automation systems is creating new avenues. These cutting-edge systems are able to help with creative processes, including content creation and strategic planning, bringing a new perspective to how companies address challenges and foster innovation.
4. Real-time data processing and edge computing
Improvements in cloud computing and edge computing are likely to accelerate the scalability and speed of automation. Based on real-time data processing, companies can instantaneously make decisions, increasing general operational agility.
5. Collaborative intelligence and enhanced decision-making
Next-generation systems will increasingly combine human insight with machine accuracy. With real-time guidance and contextual suggestions, these technologies enable teams to make quicker, better-informed decisions, foster innovation, and build a definitive competitive edge.
These trends point to the fact that the future of AI is not merely about incremental steps—it is about revolutionizing how companies do business. Businesses that remain ahead of these trends will reap the rewards of greater efficiency, more intelligent decision-making, and the capacity to rapidly respond to changes in the marketplace.
Embracing AI automation developments in the future is investing in technology that will adapt with your company. As integration with current systems becomes easier and AI models more advanced, organizations will increasingly appreciate automation. The future to invest in such developments is today, as they lay the groundwork for long-term success and strength in a competitive environment.
To sum it all up
AI automation is revolutionizing how companies compete, expand, and do business today in this rapid-paced world. Companies taking a strategic, data-centric approach are achieving greater efficiency, more intelligent decision-making, and greater agility.
By incorporating AI, companies can set themselves up to respond rapidly to evolving markets, break through operating obstacles, and fuel sustainable growth. In this guide, we have shown how AI transforms businesses—enabling predictive maintenance in manufacturing, real-time fraud detection in banking, and dynamic inventory management in retail. We have also pointed out pitfalls and included a rough outline of a simple-to-implement implementation plan.
The future for automation is bright with self-trained models, better cybersecurity, and generative AI on the horizon to reshape operations. The grand idea is that applying AI in automation isn’t merely about automating routine tasks. It’s about enhancing your decisions and future-proofing your organization. The secret to success is having a solid strategy, having quality data, getting your people aligned, and continuously optimizing your processes.
At Netscribes, we are constantly pushing the boundaries of innovation through our state-of-the-art AI-powered automation solutions aimed at empowering businesses and revolutionizing operations. Our vibrant portfolio is built on Gen AI at its foundation, allowing us to provide customized solutions that automate processes, improve decision-making, and amplify customer engagement. Are you ready to take the leap? Explore our full array of innovative AI business solutions, each designed to address the unique needs of contemporary businesses.