The future of AI in financial services: What’s next?
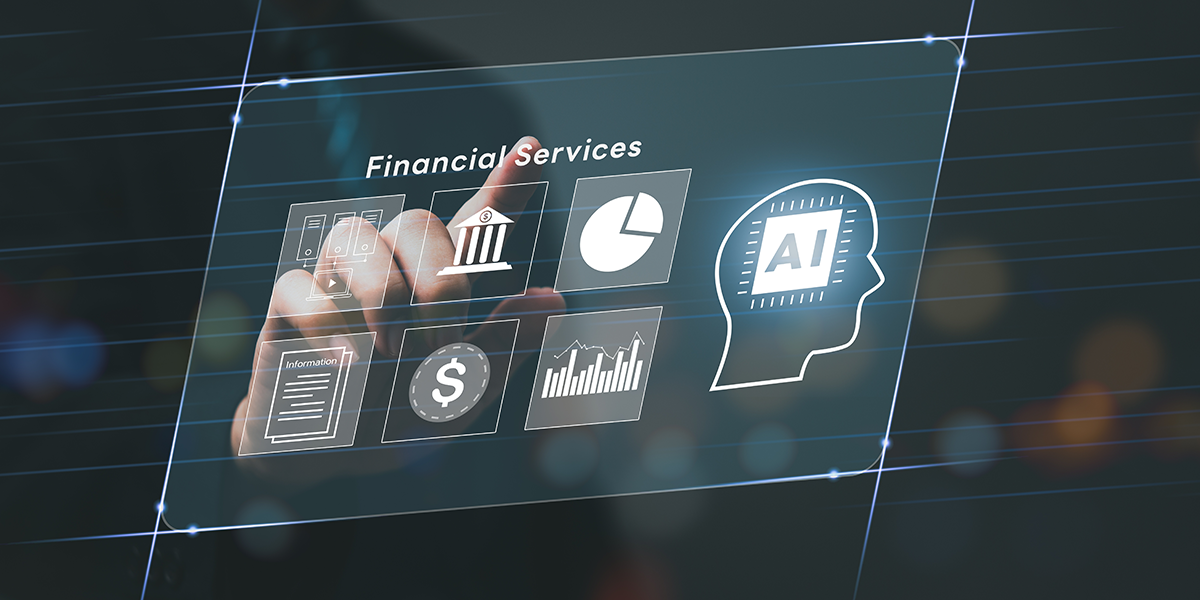
Highlights
- AI in financial services is reshaping the industry with real-time fraud detection, hyper-personalized banking, and predictive risk analytics.
- Banks are automating back-office operations using AI and RPA, reducing costs while improving speed, accuracy, and compliance.
- AI-powered payment systems and robo-advisors are democratizing access to secure, efficient, and tailored financial solutions.
- With rising regulatory scrutiny, responsible AI adoption—centered on transparency, ethics, and sustainability—is becoming a competitive necessity.
The AI-led finance shift
The finance world is changing at a pace never seen before, with booming digital channels, shifting consumer behavior, and the relentless pursuit of business agility. Artificial intelligence is, in this context, radically altering the production, customization, and delivery of financial products to end customers. Next-generation AI in financial services enables in-depth analysis of a firm’s internal and customer-related data. This automates previously manually done processes, and even creates services specific to each customer’s needs.
The outcome is a world of finance that is not limited to ticking regulatory boxes, but one that is more inclusive, real-time, and insight-driven. The direction is already manifest in the speed with which banks launch new digital offerings and the ease with which customers embrace new payment technologies and advisory platforms.
In this blog, we discuss six principal ways in which AI in financial services is driving these changes. Subjects such as fraud detection and risk management tend to dominate discussions in finance. However, AI’s influence today ranges from mundane back-office functions to complex wealth advisory services.
Fortifying fraud detection and security
Traditional rule-based fraud detection systems depend on preconfigured triggers and blacklists. These can be easily evaded by cybercriminals. Legacy systems are not flexible enough to keep pace with changing fraud methods, resulting in late detection and missed threats. AI-based models, in contrast, function in real-time. They learn continuously from transactional patterns and flag anomalies that may signal fraud. Rather than waiting for a pre-defined red flag, dynamic fraud detection systems powered by AI in financial services evaluate every transaction. They match it against historical patterns and peer group information to identify irregularities.
For example, in the case where a customer who normally makes minimal, local buys, suddenly creates a string of high-value transactions abroad, a system powered by AI recognizes right away that such activity is uncharacteristic. It may trigger a follow-up security alert, like multi-factor authentication, before it conducts the payment. This anticipatory measure minimizes false positives yet keeps fraudulent schemes from going uncaught.
Techniques to overcome sophisticated threats
Fraudsters are always adapting their techniques, and AI in financial services models have to keep pace as well. In recent years, particularly since the late 2010s, more sophisticated methods, including isolation forests and autoencoders, enable fraud detection systems to improve their accuracy. They do this by detecting outliers without the need for pre-programmed rules. These approaches mark a significant shift from the traditional rule-based systems.
Isolation forests operate by partitioning transaction data randomly and isolating anomalies that deviate from expected patterns. Since legitimate transactions have similar properties, a suspicious or fraudulent transaction is singled out much faster. This enables financial institutions to mark such activity in real time.
Autoencoders, which are a form of neural network, are also trained to reproduce typical transaction data. If a transaction generates a high reconstruction error, the system marks it for review. Since these AI models continuously update their internal parameters, they improve over time, adapting to emerging fraud patterns without human intervention.
Real-world impact and industry adoption
The financial sector has seen substantial improvements in fraud prevention through AI-driven anomaly detection. Capital One, for instance, is democratizing machine learning to enhance fraud detection within banking operations, with a focus on fraud detection, transaction intelligence, and internal innovation platforms. They’ve built an open system of tools, platforms, and capabilities accessible to all employees within business units, without the need to consider their technical credentials. By doing so, they’re making it possible for non-experts. This includes fraud analysts, operations personnel, or product managers — to experiment with and deploy ML on actual problems. This speeds up innovation, enhances results such as fraud detection, and encourages cross-functional collaboration.
With the shift from reactive to real-time prevention strategies, AI in financial services detects potential suspicious activity prior to incurring loss. Aside from savings in cost, AI-based fraud detection also takes on a critical function of protecting customer trust. False positives can alienate customers and erode their trust in a bank.
By making its anomaly detection algorithms more sophisticated and reducing unnecessary transaction holds, AI in financial services improves the entire banking process. Because fraud methods continually change, adaptive AI models operating in real time keep banks ahead of these threats, protecting both financial and reputational damages.
Crafting personalized banking experiences
Collaborative filtering functions by taking a look at how a customer spends and matching them to other people like them. If people who spend similarly are more likely to open up a high-yield savings account or put their money into a certain fund, then the system might suggest these things to you. This method assumes that individuals who share financial profiles would be interested in the same product. This thus enables banks to make personalized recommendations that seem natural and not formulaic.
Content-based filtering, in contrast, is centered on your individual history instead of group trends. If you consistently invest in renewable energy stocks, for instance, the platform will bring to your attention ESG-oriented funds or notify you of newly listed clean-tech investment options. This approach personalizes suggestions based on your stated preferences, so that financial products are matched with your proven interests instead of making assumptions about trends from wider customer behaviors.
Timely, data-driven advice can greatly improve financial decision-making. If your monthly spending suddenly increases for several months in a row, an AI-based system may recommend a budgeting app or an interest-conscious loan. Younger, digitally native consumers increasingly demand this degree of smart personalization. Through the application of AI in financial services, banks are able to transcend transactional relationships to become proactive advisory institutions that can anticipate customer needs.
Automating tasks using RPA and AI in financial services
Robotic process automation (RPA) is revolutionizing the manner in which financial institutions manage mundane work by removing repetitive tasks like data entry, compliance checks, and other manual processes. By substituting these time-wasting processes with AI-driven bots, banks can eliminate human errors, increase processing speed, and liberate employees to work on more critical tasks. Machine learning boosts RPA by adding a decision layer, especially in intricate situations. For example, if a mortgage application is in a gray area, the AI system may suggest conditional approval or refer the case to a human underwriter for further review.
Emerging technologies also optimize the productivity of automated processes. Optical character recognition (OCR) converts paper documents, including loan requests and balance sheets, into digital form and eliminates the manual entry of data. Natural language understanding (NLU) transforms unstructured text from emails, chat transcripts, and internal reports into a machine-readable format. This allows AI to automatically categorize messages and route them to respective teams. Such features optimize internal processes and customer service by routing inquiries and requests more efficiently.
The transformation to automation is reshaping financial services jobs. According to a Deloitte report, organizations scaling intelligent automation have achieved a 27% average reduction in costs. By automating repetitive administrative tasks, workers are freed up to engage in more impactful duties that call for human talent. AI in financial services is not merely about productivity. It’s about redefining priorities so that teams can concentrate on high-value work that actually drives the industry forward.
Transforming risk management with predictive analytics
Historical credit information and established default rates have been the cornerstone of traditional risk management models for years. While effective in some measure, these approaches tend to ignore larger financial tendencies, behavioral patterns, and current economic changes.
The use of this traditional approach has changed in recent years. For example, JPMorgan Chase has applied predictive models based on AI to boost its credit risk evaluation. For doing so, the firm integrates alternative sources of data like customers’ transaction history, market trends, and macroeconomic factors. This enables the bank to identify early warning signals of potential defaults and refine lending strategies accordingly. This is far beyond the capabilities of traditional credit scoring models.
Predictive analytics based on AI in financial services bring a more dynamic perspective by using varied data sources, ranging from social media sentiment to real-time commodity price movements. In contrast to static spreadsheets that must be manually updated, machine learning models realign risk parameters automatically as markets shift. For instance, if there is a big economic policy announcement overnight, AI systems can adjust lending limits within a matter of hours, keeping financial institutions nimble and responsive.
Read more: Artificial Intelligence (AI) in sentiment analysis and industry use cases
AI-driven risk management also treats several risk categories more accurately. In credit risk, AI algorithms examine both conventional credit scores and non-traditional data sources. This includes peer-to-peer lending behavior or recurring transaction patterns, to build a richer risk profile. In market risk, machine learning algorithms constantly scan trading activity and identify early warning signs of currency or equity volatility, enabling companies to forecast changes before they occur.
AI in financial services is also involved in operational risk, with it detecting inefficiency and compliance loopholes in internal procedures. If a certain approval process constantly results in regulatory mistakes, an AI audit can report the concern and recommend remedial measures before it becomes an expensive fine.
The advantages of AI in financial services for risk management go beyond internal effectiveness. AI-powered monitoring allows financial institutions to identify suspicious patterns at an early stage. This minimiizes exposure to fraudulent transactions or regulatory penalties.With real-time data analysis and predictive intelligence, AI in financial services is transforming the way institutions manage risk, making it a strategic strength rather than a reactive function.
Revolutionizing payment systems
Payment systems driven by artificial intelligence are revolutionizing fraud detection by constantly processing transactions in real-time. Mastercard announced in May 2024 that it introduced generative AI in its fraud systems. This amplified its capabilities to monitor and act on anomalies during transactions in real time. Compared to static machine learning methods, Mastercard’s generative AI has the capacity to imitate and foretell newly forming fraud schemes. This enables the system to identify suspect transactions more anticipatorily by constantly creating new fraud patterns and learning from them.
Rather than using fixed rules, contemporary systems review patterns of spending and mark down suspicious activity. For instance, if a customer who tends to make purchases at neighborhood grocery stores suddenly makes a big international transfer, AI recognizes the anomaly and triggers further verification processes. To increase security further, biometric verification techniques like voice prints, fingerprints, and facial recognition are being used more to replace passwords. This make it more difficult for imposter users to access accounts.
In addition to fraud protection, AI in financial services is also streamlining the processing of transactions. It facilitates quick and inexpensive processing of transfers by automatically selecting the most efficient payment route. If there is any delay or higher cost in terms of fees, AI shifts payments to a different route in real time. Cross-border transactions also have advantages due to the real-time processing of currency exchange by AI in financial services. This eliminate disparities and helps adhere to country-level financial compliance regulations. Automation to such a level removes the hassle of cross-border payments as well as making the transactions highly reliable across the globe.
The advantages of AI-based payment systems benefit customers and financial institutions alike. AI cuts down on fraudulent transactions considerably while speeding up genuine payments. Such a seamless experience prompts customers to embrace digital payment solutions. This ultimately leading to higher transaction volumes and revenue for banks. Through the use of AI in financial services, institutions can build a more secure, smoother, and highly interconnected global payments environment.
Democratizing financial advice with robo-advisors
Robo-advisors are revolutionizing investment by making financial advice easier, automated, and customized. Historical investment methods tend to involve in-depth research or taking advice from a financial advisor. But AI-driven robo-advisors eliminate the need for guesswork in portfolio management. The systems evaluate a user’s risk tolerance in terms of expenditure patterns and financial aspirations. It ensure that investment suggestions are in sync with their comfort zone.
For instance, a user who consistently spends a lot of money on a purchase may have the system infer higher risk tolerance and recommend a more aggressive portfolio. Robo-advisors also manage ongoing portfolio management, rebalancing asset allocations automatically with changes in the markets. Rather than needing to have users watch stock indexes every day, such systems maintain portfolios, even in times of economic uncertainty.
One of the largest benefits of robo-advisors is the way they encourage investing among more people. Financial advisors tend to be expensive, thus professional investment management is a possibility only for high-net-worth individuals. Robo-advisory services, by contrast, provide an affordable solution. The onboarding process itself is user-friendly. It helps users navigate investment vehicles with informative recommendations and straightforward instructions. From saving for retirement to accumulating wealth, the process is streamlined to eliminate the complexity typically surrounding bonds, stocks, and mutual funds.
AI in financial services can also potentially increase wealth-generating opportunities, especially for middle-income individuals. By serving a wider customer base, banks are able to diversify their customer base and enhance brand loyalty. It also gets more individuals to engage actively in investing instead of keeping their money idle. As more users interact with these platforms, financial literacy enhances, resulting in better, well-informed investors. AI-driven robo-advisors can thereby transform how people think and interact with their financial futures.
The way forward: flexibility, ethics, and regulation alignment
AI in financial services is certainly shaking up the sector, but regulators aren’t sitting on the sidelines. Around the world, data privacy and algorithmic fairness are under greater scrutiny. Within the European Union, the GDPR is the gold standard for data protection, forcing companies to explain how they collect, store, and use customer data. Other places are catching on, legislating against discriminatory AI models and stopping invasive data practices. For banks, getting in front of these regulations isn’t merely a matter of staying out of trouble. It’s actually about creating systems that clients can rely on.
The best banks don’t view these regulations as obstacles. Rather, they leverage them as chances to rationalize processes, creating systems driven by AI in financial services that are future-ready. By creating compliance-first architectures, banks and other financial institutions can develop real-time data monitoring, transparent decision-making frameworks, and robust governance systems. By doing so, they not only remain ahead of changing policies but also minimize the risk of surprise regulatory issues. Tools in AI in financial services can even monitor compliance on their behalf, highlighting potential issues before they become expensive problems.
Compliance goes beyond checking boxes for regulators; robust compliance fosters customer trust. Consumers need to know their money is being handled responsibly. They will be more willing to use AI in financial services if they can trust the institutions offering them. Banks that lead with transparency, equity, and security will not only avoid regulatory issues. Customers who care about trustworthy AI will deepen relationships with them. In a business based on trust, that’s a strategic advantage any financial institution cannot afford to ignore.
Read more: AI in stock market: Enabling smarter trading, forecasting, and investment strategies
Conclusion
AI in financial services is transforming the industry, from instant fraud defense to dynamic product updates. Organizations that effectively leverage AI gain significant advantages in operational efficiency, customer experience, and regulatory compliance. True transformation is not just merely the deployment of new technology. It involves building a resilient form of AI in financial services that is intelligent and ethical in an ever-evolving environment.
As banking continues to expand its use of AI, it will increasingly overlap with blockchain, quantum computing, and decentralized finance. This drives a shift towards more data-based, interconnected global banking models. Customers will benefit from sophisticated and accessible financial products. Institutions that are able to manage risk alongside innovation will set the industry benchmark for best practice.
But the take-up of AI in financial services has to be carefully managed. Bias in algorithms, cyber risks, and over-reliance on automation remain pressing concerns. Banks must have in place a strong governance framework and compliance mechanisms to ensure that AI-enabled innovation does not compromise on trust. The future of finance is not about smarter algorithms. It’s about harnessing technology with human acumen to create a safer, more transparent, and customer-centric financial system.
From AI strategy consulting to machine learning models tailored to your needs, Netscribes assist financial institutions in implementing AI responsibly and efficently. Our experience guarantees smooth integration, high-performing models, and adherence to industry regulations—so that you can concentrate on providing secure and data-driven financial services. Discover our AI in financial services to learn how we can enable your organization to open up new possibilities with trust, transparency, and lasting success.