Automated data cleaning in finance: A smarter way to tackle fraud and risk
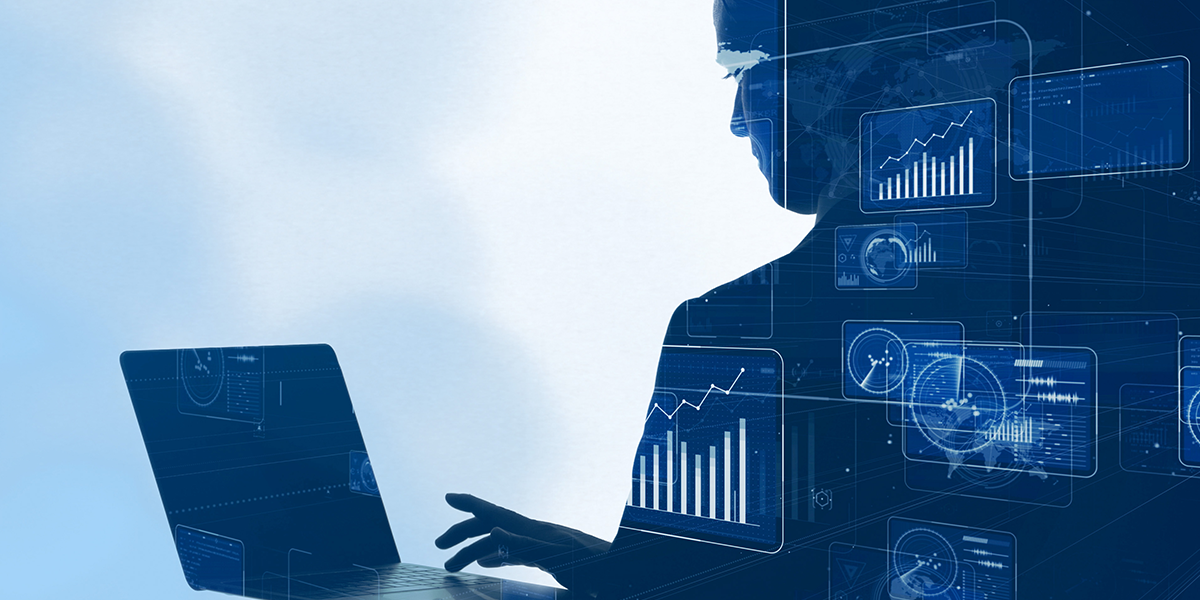
Highlights
- Clean data is crucial for fraud detection, risk management, and regulatory compliance.
- Manual methods can’t keep up with today’s data volume—automation and AI are now essential.
- AI tools improve accuracy by spotting anomalies, syncing records, and validating data in real time.
- Reliable data enables faster decisions, fewer errors, and stronger customer trust.
At first glance, data cleaning might seem like a routine task—maybe just fixing typos or adjusting formats. In reality, however, making sure your data is consistent and reliable is essential for uncovering fraud, managing risk, and staying compliant with ever-changing regulations. When financial institutions fail to keep databases accurate, they risk serious liabilities, ranging from missed red flags in fraudulent transactions to uncomfortable conversations with regulatory bodies. Fortunately, new technologies like automation and artificial intelligence (AI) are reshaping how organizations handle this process.
In the sections below, we will explore why data cleaning is so critical in today’s finance world, how advanced tools are modernizing these efforts, and which steps firms can take to remain one step ahead of fraudsters and compliance pitfalls.
The domino effect of unreliable data
Financial institutions rely on precise data for tasks including but not limited to loan approvals, investment portfolio assessments, and fraud detection. The problem is that errors accumulate easily. You might have outdated customer addresses, duplicate client records, or missing entries in transaction logs. Any of these hiccups can create a domino effect that disrupts core operations.
Misplaced fraud flags
When different parts of your organization maintain contradictory information, there is an increased likelihood to overlook or misinterpret potential fraud indicators.
Weak risk assessments
Risk models demand accurate data to forecast scenarios like default risks, market swings, or liquidity constraints. Even minor inconsistencies can undermine those projections, leading to flawed strategic decisions.
Compliance troubles
Financial regulations—from know your customer (KYC) to anti-money laundering (AML)—impose strict rules on verifying and retaining customer information. Missing data points or conflicting records can trigger penalties and dent your institution’s credibility.
Customer friction
Mistakes in recordkeeping can inconvenience legitimate customers. For instance, if someone’s address isn’t updated properly, they may fail a security check or miss important mailings.
Delaying or ignoring the need for clean data won’t just affect individual departments; it can ripple throughout your entire organization.
Moving beyond manual methods
Historically, many banks and investment firms have tried to handle data cleaning by hand. Analysts sift through massive spreadsheets or legacy databases, spot inconsistencies, and correct them. This manual approach quickly becomes unsustainable, especially considering the volume and velocity of modern financial data. Online transactions, mobile deposits, and real-time trading produce streams of information that humans simply can’t review in a timely manner.
Scale
The sheer number of daily transactions or account updates makes it impossible for teams to keep up using only manual methods. Even small errors can slip by unnoticed, potentially leading to considerable risks.
Consistency
When employees manually fix errors, standards can differ. Each analyst might have a slightly different approach to labeling or formatting data, resulting in inconsistencies down the line.
Timeliness
Data quickly becomes stale. By the time a quarterly cleanup is finished, new errors may have already piled up. Real-time solutions can detect issues as they emerge.
Value of human capital
Automating large portions of data cleaning tasks frees employees to focus on higher-level work, such as refining risk models or enhancing customer experiences.
Modern financial services increasingly look to automated solutions for data cleaning, not just to save time but also to ensure accuracy and consistency at scale.
AI takes data cleaning to the next level
Automation, on its own, can handle repetitive data tasks—such as standardizing date fields or merging duplicate entries. However, the incorporation of AI can make data cleaning far more dynamic. Machine learning algorithms adapt over time, allowing them to catch subtle anomalies that simpler scripts might miss. Below are some core AI-driven methods that streamline the process.
Machine learning for fraud detection
- Advanced algorithms analyze huge datasets to spot unusual transaction patterns or suspicious activity.
- These tools continuously learn and update their fraud thresholds, becoming more effective at data cleaning as they ingest new information.
Robotic process automation
- RPA bots can synchronize customer records across multiple internal platforms—like payment systems, loan approval software, and CRM tools.
- By removing duplications and mismatches, they improve the accuracy of daily operations.
Natural language processing
- NLP extracts vital data from unstructured sources such as customer emails, PDF forms, and chat conversations.
- Once that data is converted into a consistent format, it becomes much easier to integrate with existing systems.
Automated deduplication and entity resolution
- After mergers or system migrations, it’s common for institutions to hold redundant records for the same individual or entity.
- These automated tools can consolidate duplicates, making risk assessments and fraud checks more accurate.
Real-time data validation
- Rather than waiting for scheduled audits, real-time validation detects and corrects errors the moment they appear.
- This ensures that data remains current and eliminates blind spots before they escalate into bigger problems.
By using AI to automate much of the data cleaning process, financial institutions can maintain higher data quality while also reducing the workload on their human teams.
Read more: How AI and data analytics are redefining insurance fraud prevention
Fraud prevention depends on data cleaning
Modern criminals employ a variety of methods—from synthetic identities to phishing schemes—to exploit weak spots in financial databases. If your data is incomplete or disorganized, you are effectively handing over an advantage to fraudsters. This is where ongoing clean data plays a defensive role.
Clear fraud signals
- High-quality data helps flag anomalies quickly, ensuring that suspicious transactions or login attempts get escalated promptly.
- Unclean data, by contrast, can lead to overlooked red flags and missed signals of unlawful activity.
Fewer false positives
- A well-maintained database reduces cases where ordinary customer actions are incorrectly flagged as suspicious.
- This streamlines investigative work, allowing fraud analysts to center their attention on genuine threats.
Faster resolution
- When investigators have to piece together an incident across multiple, unlinked data sources, time is wasted.
- Consolidated and accurate records speed up the entire investigation, minimizing the window in which criminals can operate.
Fraud prevention is, therefore, tightly intertwined with data cleaning. By making sure your records are consistently up to date, you can spot unusual behavior faster and keep your institution’s resources safeguarded.
Towards more informed choices
Although fraud prevention grabs headlines, data cleaning also plays a huge part in broader risk management efforts. From predicting loan defaults to analyzing overall market vulnerabilities, accurate data is crucial.
Credit risk: A borrower’s application hinges on numerous data points—income, credit history, existing liabilities. If that data is incomplete, your decisions become guesswork.
Market risk: Trading and investment desks need timely, correct data on market trends, trading volumes, and historical performance. Any discrepancy can result in inaccurate positions or hedging strategies.
Operational risk: Errors in data bring about extra administrative costs, compliance slip-ups, or delays that harm an institution’s operational efficiency.
Regulatory compliance: Agencies such as the SEC, FCA, or local banking authorities demand precise recordkeeping. Regular data cleaning helps you stay aligned with those expectations.
Reliable data is the cornerstone of well-founded judgments in finance, guiding everything from everyday tasks to strategic decisions about products, services, and markets.
Make clean data your competitive advantage
In the world of finance, even a small data mistake can escalate into a serious setback—whether that’s a hidden fraud vulnerability or regulatory sanctions. By prioritizing data cleaning, your organization can turn jumbled, inaccurate information into a treasure trove of insights that sharpen risk detection, bolster customer trust, and streamline internal processes.
Relying on purely manual methods has become impractical in today’s fast-paced digital environment, making technology adoption essential for maintaining data quality at scale. If you’re looking for cost-effective customized services in the market, you can check out Netscribes’ AI business solutions to enhance data accuracy and drive business growth.