Machine learning solutions in healthcare: driving precision medicine and patient care
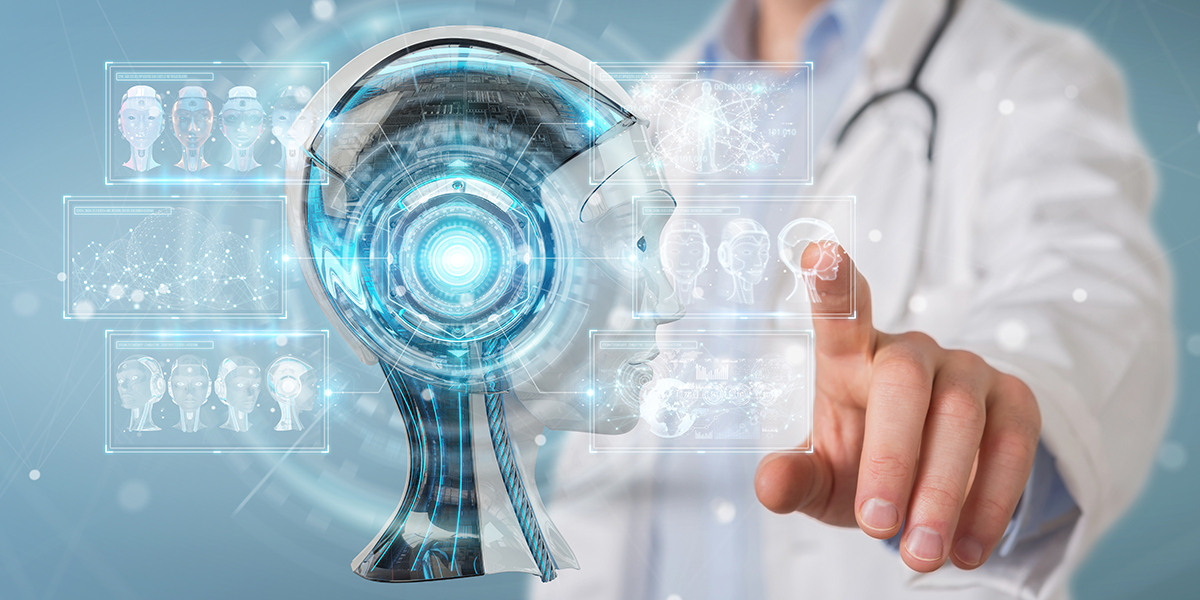
Highlights
- Machine learning is improving diagnostic accuracy, with neural networks detecting early disease signals invisible to the human eye—accelerating detection and improving patient outcomes.
- Personalized medicine is going mainstream, as AI matches treatments to genetic profiles, predicts drug response, and optimizes dosage for individuals at scale.
- AI is slashing drug development timelines, helping teams repurpose existing drugs, design smarter trials, and bring critical therapies to market faster.
- Predictive analytics and automation are transforming care delivery, from forecasting hospital readmissions to streamlining scheduling, claims, and EHRs.
A new era of healthcare
In medicine, where seconds make a difference and the margin for error is paper-thin, the pressure to be more accurate, productive, and precise has never been greater. For example, delays caused by misdiagnosis of conditions like cancer or orphan diseases continue to affect patient outcomes and burden healthcare systems. In parallel, the exorbitant costs of drug development present a significant impediment to getting new treatments to the market. But the application of a machine learning solution promises to be just the tool that can overcome such challenges.
Traditional rule-based systems in medicine were constrained to automating simple tasks by set instructions. However, today’s machine learning systems are significantly different. They extend far beyond the realm of automation. They learn from large data sets, find subtle patterns, and improve progressively with new data input. In this way, adaptive intelligence is injecting fresh life into healthcare processes.
Read on to explore the five key ways machine learning solutions are transforming the healthcare industry.
1. Neural networks that spot what humans miss
AI-driven tools can now spot the earliest signs of tumors or microcalcifications in mammograms. The accuracy surpasses that of seasoned radiologists. This is one of the most exciting applications of applying a machine learning solution in healthcare as it significantly boosts diagnostic precision. There are now several deep learning machines that are entirely automated and rely on convolutional neural networks for determining breast density. According to research, an externally validated algorithm demonstrated an 89% accuracy in stratifying dense and non-dense breasts, and a 90% agreement rate between three independent readers and the algorithm.
Ever since the early 2010s, especially after CNNs became more popular following the success of AlexNet in 2012, these models have increasingly been utilized in medical imaging. They are being trained over large datasets to pick up on faint patterns that can be overlooked by the human eye.
In traditional practice, diagnosing a complex disease often hinges on a physician’s ability to interpret medical scans, lab results, and patient histories. Contrastingly, deep learning models, especially convolutional neural networks (CNNs), excel at image recognition tasks. By training on thousands or even millions of medical images, these models learn to identify subtle indicators that might be missed during a manual review.
The application of a machine learning solution also extends beyond imaging. Genomics-based diagnostics use algorithms to sift through a patient’s genetic data, flagging markers associated with hereditary conditions. This allows for earlier interventions or preventive measures.
2. Custom care at scale: individualized treatment programs
Besides enhancing diagnostic accuracy, there has also been a substantial shift from one-size-fits-all medicine to personalized medicine. In the 2010s, with the rise of big data and machine learning, personalized medicine accelerated. This is especially relevant to oncology, where personalized treatments started overtaking more nonspecific treatments.
For example, a model could find that patients with a particular genetic defect respond particularly well to one type of chemotherapy agent, but other patients with varying biomarkers respond better to another treatment option. Being aware of this beforehand saves patients from unneeded side effects, reduces trial-and-error practices, and maximizes overall treatment effectiveness.
Rather than writing the same prescription for all patients, physicians now access machine learning models trained from varied data sets. This includes electronic health records (EHRs), laboratory results, real-time patient tracking, and even lifestyle information.
By comparing these data sets, computers can forecast the way various patient subsets would react to certain therapies. The machine learning solution aids in dose optimization as well. By incorporating parameters such as organ function, genetic markers, and patient weight, predictive algorithms are able to project the optimal dosage for an individual. This cuts down on negative reactions and optimizes clinical results—a gain for healthcare systems and patients alike.
3. Drug development: How AI compresses a decade into days
The drug development pipeline is notoriously time-consuming and expensive. It often takes billions of dollars and more than a decade to progress a compound from laboratory tests to clinical deployment. At the beginning of the COVID-19 pandemic, conventional drug discovery timelines were a huge challenge. In response to this, the UK-based AI firm BenevolentAI employed a machine learning solution to quickly scan for potential drugs by examining known drugs that can be repurposed.
Their system analyzed enormous quantities of biomedical information. This included scientific papers, clinical trials data, and chemical properties of drugs approved by the FDA. The purpose? To find compounds that could potentially offset the virus’s effects. The platform identified baricitinib, a drug for rheumatoid arthritis, as a potential candidate with antiviral and anti-inflammatory activity within a few days.
Based on this AI-generated insight, scientists launched clinical trials much more quickly than normal. Baricitinib ultimately became one of the medications approved for emergency use in the treatment of hospitalized COVID-19 patients. It greatly reducing the discovery-to-deployment timeline.
Machine learning solution applications also increasingly help in the optimization of clinical trial design. For example, an algorithm can pinpoint patient subgroups who will benefit most from a novel treatment, directing the inclusion criteria. This makes the process more cost-effective and faster because the trials become more focused and deliver more decisive outcomes. In a few instances, even adaptive clinical trials permit analysis in real-time. The parameters of the trial (dosage, patient inclusion criteria, etc.) change in accordance with new data.
4. Reducing readmissions with predictive analytics
While machine learning is revolutionizing drug discovery, its effect reaches far into patient care. One of its most notable applications is to predict clinical outcomes before they take place.
A particularly striking example is from a 2019 paper in The National Library of Medicine, where authors developed a machine learning model to forecast the risk of 30-day readmission to hospital for patients with heart failure based on retrospective electronic medical records data. The model outperformed conventional logistic regression techniques by a significant margin. This provided a more detailed picture of patient risk by making use of high-dimensional clinical data.
As opposed to traditional scoring models based on a restricted set of factors, a machine learning solution can accept heterogeneous sources of data. These prognostic algorithms draw information from many sources—vital signs, medication refill records, laboratory tests, and even socioeconomic status. The outcome is a risk score that reflects how likely a patient is to be readmitted in a certain timeframe. A patient who is flagged as high-risk may get additional home health visits, remote monitoring equipment, or more frequent telephone follow-up calls. This can steer minor problems from becoming major complications.
This transition from reactive care to predictive, data-led management is part of a broader transformation in healthcare, one in which AI enables clinicians to act ahead of complications, enhancing results and relieving pressure on health systems.
5. Redefining efficiency through precision automation
It’s easy to emphasize the life-saving uses of machine learning applications. But there is also immense opportunity in optimizing the administrative processes in healthcare. Some applications include:-
- Automated scheduling and chatbots: Routine questions—such as appointment reminders or symptom screening—can be delegated to AI-based chatbots. The chatbots utilize natural language processing (NLP) to analyze patient queries and provide pertinent answers. Staff then have more time for difficult questions that really do need human touch.
- Claims processing and billing: A machine learning solution can double-check billing codes, check insurance eligibility, and even estimate the probability of a claim being approved. Flagging out-of-the-norm issues up front decreases delays and lessens rework later on.
- Electronic health record optimization: NLP algorithms are capable of scouring out long EHR notes, extracting critical details like diagnoses, prescriptions, and lab results. This not only accelerates data retrieval but also allows for accurate, current patient records to be maintained.
Read more: The future of AI and big data in healthcare: What to expect by 2030
The path forward
As machine learning solutions improve, opportunities in the healthcare industry promise to be exciting and pragmatic. We already have swift improvements in diagnostics, tailored treatment, and hospital operating efficiency. The next step could entail greater collaboration with wearable devices, more sophisticated real-time analysis for clinical decisions in the moment, and increasingly dynamic clinical trial methods.
Nevertheless, there are challenges. Managing data quality, sustaining strong patient privacy requirements, and dealing with regulatory structures will need to be carefully planned and coordinated. Healthcare is an area where any mistake can lead to adverse outcomes. Testing and regularly checking machine learning models is thus imperative.
Finally, no machine learning solution is about replacing doctors, nurses, or pharmacists. Instead, it’s about increasing their capacity for delivering high-quality, patient-oriented care. Through collaboration with these models, healthcare professionals can better understand large volumes of data, identify key trends, and make evidence-based decisions. This translates into patients receiving more accurate interventions, fewer surprises, and better overall health outcomes.
At Netscribes, we work with life science and healthcare firms to improve processes and enhance research through artificial intelligence-based solutions. Discover our AI business solutions and learn how we’re empowering businesses to turn tough data into powerful insights to fuel smarter, speedier healthcare decisions.