Can AI be eco-friendly? The rise of sustainable solutions for AI in the digital era
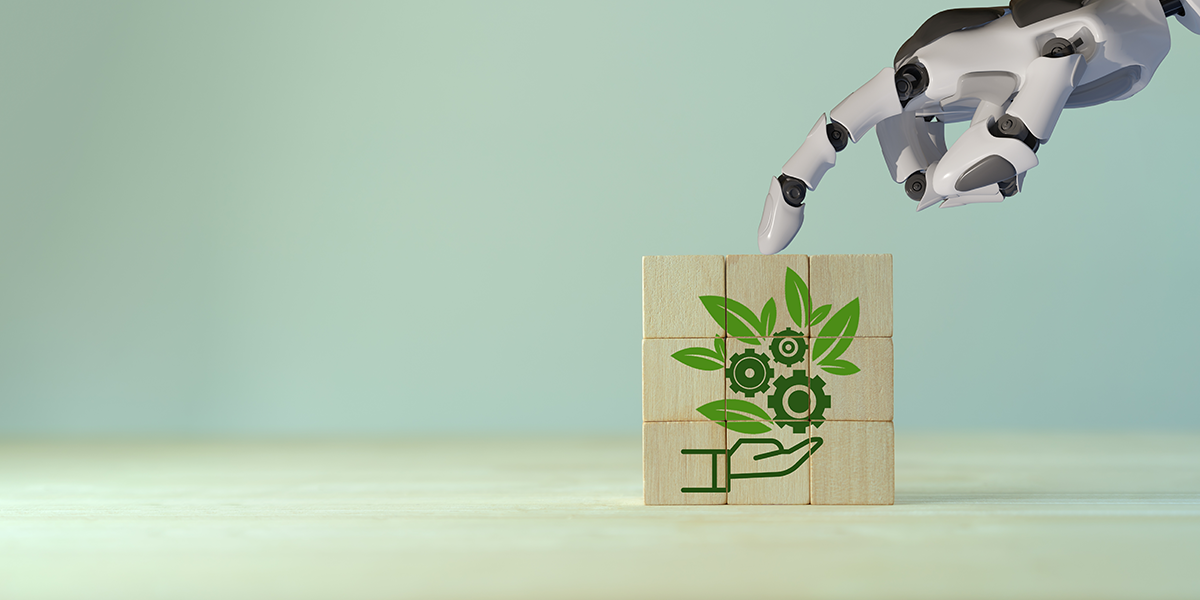
Highlights
- AI’s environmental cost is rising fast — with data centers and model training consuming massive energy, the push for greener AI has never been more urgent.
- Smarter models and leaner architectures like Google’s EfficientNet show that high performance doesn’t have to mean high energy consumption.
- Companies are rethinking infrastructure — using liquid cooling, renewable energy, and edge computing to power AI sustainably at scale.
- Sustainable AI is a long-term advantage — reducing costs, aligning with regulations, and driving responsible innovation across industries.
The AI boom faces a climate reckoning
Artificial intelligence is transforming the way we work and live, from the virtual assistants in our pockets to the sophisticated algorithms driving big business decisions. But behind this digital revolution lies a rising environmental issue. The huge data centers that power AI are consuming mammoth amounts of energy—so much so that cloud computing’s carbon footprint is larger than the airline industry’s. It’s a paradoxical dilemma: the same technology propelling us forward is also straining our planet. But what if technology could be a part of the solution rather than the problem?
In this blog, we explore the emergence of sustainable solutions for AI—delving into the innovations that are making artificial intelligence greener and leading the way to a more sustainable digital world.
The environmental footprint
AI is revolutionizing sectors and mapping the future, but there’s a secret cost that most do not realize—the environmental cost. Researchers at OpenAI discovered that the computing needed to train leading AI models has been doubling every approximately 3.4 months since 2012—creating a runaway growth in energy demand. Each AI application or chatbot is built on a huge infrastructure that requires colossal amounts of power and resources. The more sophisticated AI models become, the greater the energy and power required to train and operate them.
Such meteoric expansion carries severe environmental ramifications, demonstrating the need for sustainable solutions for AI practices. Data centers powered by AI already account for enormous electricity use, not only for calculations but also for keeping them cool, which contributes to their carbon usage. Forecasts indicate that as early as 2040, ICT emissions alone might account for 14% of total emissions, with most coming from supporting infrastructure for AI. And it’s not only about energy—developing AI machines demands special hardware, and that implies rare earth mining, producing electronic waste, and even local water supplies for cooling.
AI can be used to address global issues, but its fast growth has a cost we cannot afford to overlook. One study even estimates that by 2027, the AI sector may use as much energy as a nation the size of the Netherlands. With such mind-boggling estimates, it is not just a prudent decision to move towards sustainable solutions for AI; it is an exigent demand.
Why care about sustainable AI
Given AI’s significant impact on the environment, the drive for sustainable solutions for AI is really picking up steam. Not only does it help the planet, but it makes good business sense. Energy-efficient AI saves electricity and reduces cooling costs. This boosts bottom lines while reducing carbon footprints. When consumers, investors, and regulators are increasingly calling for more environmental responsibility, being a sustainability leader is a compelling competitive edge.
In addition to the business benefits, sustainable AI is also in line with the United Nations Sustainable Development Goals (SDGs). It offers a responsible innovation framework that addresses global problems while causing the least amount of damage to the environment. With governments imposing stricter regulations on emissions and energy consumption, businesses that embrace sustainable AI practices today will be at an advantage. They will also prevent expensive compliance headaches in the future.
But the effects of sustainable solutions for AI extend even deeper. When companies invest in more sustainable AI, those same sustainable values tend to bleed over into other areas of their business. This creates greater change across sectors. Sustainable AI is not only about improving AI for the world. It’s about rethinking how technology as a whole can move toward a more responsible, efficient and sustainable future.
Key areas for implementation
Developing genuinely sustainable solutions for AI involves more than simply reducing energy usage. It demands a transformation in how AI is constructed, implemented, and powered at each level. There are four major areas where AI can be made greener without sacrificing performance.
1. Smarter, leaner AI models
AI need not be energy-intensive to function. By optimizing the design of models, engineers can significantly reduce energy consumption without compromising precision. Methods such as neural architecture search (NAS) assist in finding efficient model architectures that need less processing capacity.
For example, Google researchers launched EfficientNet, a model family found using NAS that reached state-of-the-art ImageNet accuracy using an order of magnitude fewer FLOPs (floating point operations) than prior models. By scaling architecture more wisely, EfficientNet significantly lowers energy consumption and hardware needs, creating a benchmark for more sustainable AI. In real-world implementation, these fine-tuned models are deployable on edge devices, obviating the requirement of continuous cloud computation and diminishing the environmental footprint.
2. Sustainable hardware and infrastructure
AI is based on strong hardware, but not all processors are the same when it comes to energy efficiency. Moving to AI-specialized accelerators such as Tensor Processing Units (TPUs) or special GPUs can reduce power consumption. Additionally, conventional air-based cooling is energy-hungry, but liquid immersion cooling can cut usage by as much as 50%. Where data centers are situated also makes a difference—locating them in naturally cooler regions or where there is easy access to renewable energy sources can help reduce their carbon footprint.
3. Renewable energy powered AI
The AI sector is one of the largest users of electricity, so the incorporation of renewable energy sources is a game-changer. Microsoft collaborated with Vattenfall, a Swedish utility company, to power its Sweden-based data centers with 100% renewable energy, including those that support AI model training and Azure-based ML services. The data centers are coupled with Vattenfall’s 24/7 hourly matching solution, which monitors hourly renewable energy availability in real time and matches energy usage. This configuration enables Microsoft to dynamically schedule AI workloads during times of maximum wind or hydropower availability, so that energy-intensive training jobs are performed with the least environmental footprint.
Firms are putting money into solar, wind, and hydroelectric power to power their AI hardware more responsibly. But the energy source isn’t the only consideration, timing is important as well. Smart workload scheduling allows for energy-hungry AI tasks to be executed when renewable energy generation is highest. Not only does this efficient strategy minimize fossil fuel dependency, but it also enables firms to reduce operating expenses.
4. A full-circle approach to AI sustainability
Sustainable solutions for AI do not merely centre around how AI runs—it’s also about what happens before and after its lifecycle. From responsibly sourcing raw materials for hardware to recycling outdated AI chips, every phase of AI production has an environmental impact.
NVIDIA, a top manufacturer of AI hardware (GPUs), has started to incorporate circular economy thinking into its hardware life cycle. The company procures materials like copper, aluminum, and rare earths with increasing focus on sustainable mining practices and supplier sustainability certifications. In manufacturing, NVIDIA strives to minimize waste and water consumption through certified clean production facilities.
In the end-of-life phase, NVIDIA takes part in take-back electronics schemes and has brought to market refurbishment and resale programs for preowned GPUs—particularly in data center environments with heavy AI hardware replacement. Such refurbished devices are redirected to lower energy consumption applications or research environments to prolong the use of hardware while minimizing electronic waste.
NVIDIA also discloses Scope 3 emissions, which include the environmental impact of raw materials and product end-of-life, and is aligning with GHG Protocols and Science-Based Targets Initiative (SBTi) in order to remain accountable.
Like NVIDIA, organizations are beginning to adopt circular economy principles, designing AI systems with long-term sustainability in mind. Standard-setting bodies are also working on guidelines to measure and improve AI’s environmental footprint, helping companies stay accountable for their impact.
Technical advances that will allow greener AI
The drive towards sustainable solutions for AI is not merely about solutions for consuming less power. It is about rethinking the whole system to be more efficient right from the ground level. From more intelligent models to advanced hardware, there are a number of breakthroughs that are transforming the way AI systems function while reducing their carbon footprint.
More intelligent AI models with reduced usage
AI models do not have to be large to be potent. Experts are working on methods that enable AI to be just as accurate but use much less computing power. Quantization scales back the mathematical precision in neural networks, which reduces memory needs and saves energy. Weight pruning eliminates unneeded connections in AI models, making performance leaner without compromising accuracy. Knowledge distillation enables smaller models to be trained from larger, more complex ones, still having their intelligence while functioning with much fewer resources. These developments enable sustainable solutions for AI, without making them any less effective.
Hardware that is energy-efficient
AI workloads require more than raw computing power. They require hardware capable of taking complex operations in stride without burning up loads of energy. Standard processors fall behind, using many times the power required for AI-based tasks. That is where special accelerators enter the picture, specifically optimized to process machine learning workloads but use a lot less energy.
Outside of TPUs and ASICs, neuromorphic computing is also looking to change the game. Inspired from the workings of the human brain, this strategy employs artificial neurons and synapses to process information with biological efficiency-like mimicry. The outcome? AI systems that use a fraction of the energy needed by traditional architectures and offer stunning processing abilities. As AI moves into an increasing number of applications from autonomous systems to edge computing—energy-efficient hardware is no longer a nicety. This will be the ultimate key to enable sustainable solutions for AI and make it truly intelligent.
Edge computing for localized AI processing
The majority of AI calculations take place in massive data centers that need huge amounts of energy for computation and data transfer. Edge computing lessens the load by transferring AI computing nearer to data generation sites. Rather than transferring huge volumes of information to the cloud, edge equipment handles data locally. This lessens data traffic and decreases energy consumption. Edge computing is particularly helpful for internet of things devices, autonomous cars, and smart infrastructure, where unceasing data transmission would involve high energy needs.
Decentralized AI training using federated learning
The conventional AI models need to send huge quantities of data to centralized servers in order to be trained, and this takes up energy and invades privacy. Federated learning addresses this challenge by enabling AI models to be trained on the local devices. Rather than transmitting raw data to a global server, the model itself is distributed to local devices, learns from their data, and collectively updates. This approach diminishes data transfer needs considerably while protecting security and efficiency. Federated learning illustrates how AI can be both sustainable and privacy-aware.
These innovations are empowering sustainable solutions for AI. As research continues, AI systems will become even more efficient, proving that advanced computing and environmental responsibility can go hand in hand.
From vision to action
Developing sustainable solutions for AI is not simply a matter of adjusting a few parameters or going green with the energy used. It also involves a change of culture where sustainability becomes an integral part of an organization’s DNA. Industry leaders can’t make sustainability an afterthought. They need to integrate it into each phase of AI development, from design to deployment. Transparent governance frameworks, quantifiable sustainability targets, and accountability across all levels guarantee that green AI is not merely a catchphrase but a business imperative.
A strong technical foundation is just as important. Investing in energy-efficient hardware, optimized data centers, and smart monitoring systems can significantly cut AI’s environmental footprint. Choosing vendors with strong sustainability practices and prioritizing energy-efficient equipment might require an upfront investment. However, these choices often lead to long-term cost savings while reducing carbon emissions.
After all, change doesn’t always happen overnight. It’s not easy for many organizations to transition to sustainable solutions for AI. Training programs and education are what educate teams about the effect of AI on the environment and provide them with methods to reduce it. Leadership is also crucial. When leadership makes sustainable solutions for AI a priority and recognizes teams for green achievements, eco-friendliness becomes company culture instead of something that’s occasionally done.
Read more: Sustainability technology trends: Innovations in green development for a sustainable future
The future of sustainable solutions for AI
As the environmental footprint of AI becomes more visible, global regulations and standards are emerging. Global institutions such as the International Electrotechnical Commission (IEC) and International Organization for Standardization (ISO) are creating standards to quantify and enhance the sustainability of AI. Such standards will encourage companies to apply standard methodologies for monitoring energy consumption, raw materials, carbon footprint, and waste management. Companies who act proactively today will be more equipped to address future legislation while establishing themselves as competitive entities.
Aside from policy, AI efficiency research is also changing fast. Scientists are developing new machine learning that uses less computational power, mimicking the energy-saving processes of nature (biomimicry). There is also hope with the emergence of quantum computing, capable of solving vexing AI problems while using much less energy than regular computing.
No single company can approach sustainable solutions for AI alone. Collaboration across industries, academia, and policymakers is key. Open-source initiatives are sharing energy-efficient algorithms. Industry consortia are developing best practices. Cross-sector partnerships are driving innovation. These efforts recognize that AI’s environmental impact is a shared challenge, and solving it requires collective action.
Conclusion
The move toward sustainable solutions for AI isn’t simply for the good of the planet. Businesses that focus on efficiency in the creation and deployment of AI are not only reducing energy expenses and enhancing operational efficiency, but also creating a future-proof reputation that appeals to increasingly green-conscious customers and regulators alike. As sustainability becomes a key pillar of business decision-making, AI that has a reduced footprint will not only be desirable—it will be demanded.
The benefits of sustainable solutions for AI extend beyond corporate reputation and regulatory compliance. More efficient, optimized AI models slash processing power requirements, decrease cloud computing expenses, and prolong hardware life, making both financial and environmental gains. As rules get stricter and consumers call for accountability, companies that incorporate sustainable solutions for AI now will be in a much better place tomorrow.
At Netscribes, we recognize that AI is only as good as the strategy behind it. Whether you’re streamlining machine learning models for performance, implementing sustainable solutions for AI in your business practices, or getting ready for the next generation of green tech regulations, our experience means that your AI innovation is as responsible as it is innovative. Check out our AI business solutions to explore how we can help you automate systems with intelligent algorithms.