Why the data cleansing process is critical for business success
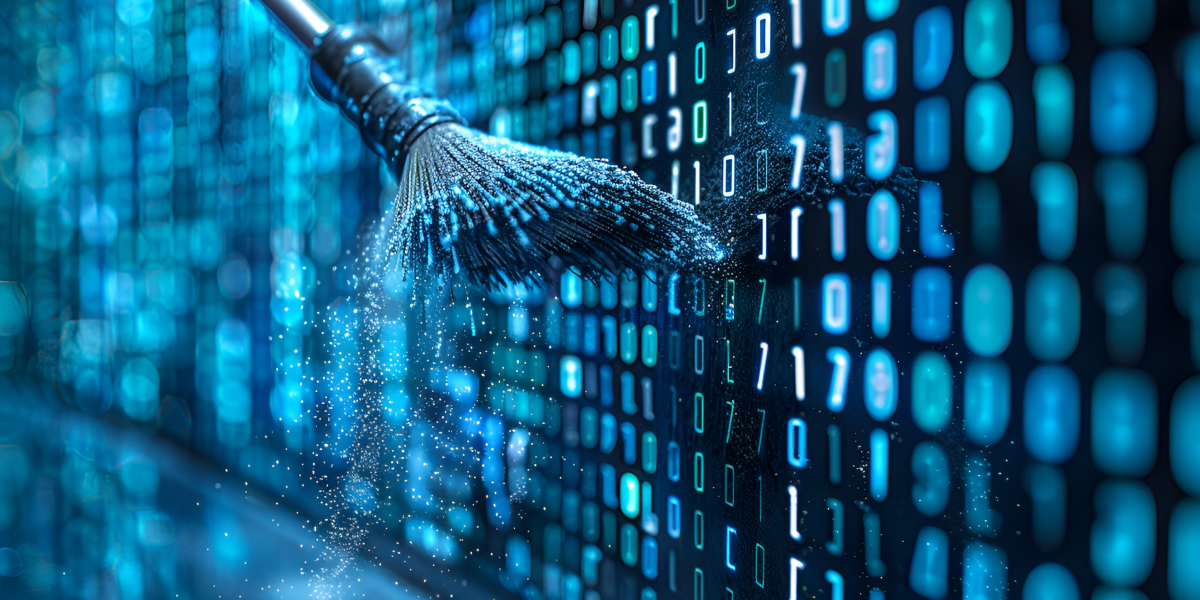
Highlights
1. Poor data quality costs businesses millions annually in lost opportunities and inefficiencies.
2. The data cleansing process detects, corrects, and removes errors for accurate decision-making.
3. Clean data enhances customer relationships through personalized interactions.
4. Regulatory compliance requires accurate data to meet GDPR, CCPA, and other standards.
5. Automating data cleansing improves efficiency and reduces manual effort.
6. AI-driven tools are revolutionizing data quality management with predictive maintenance.
In the data-driven world, businesses thrive on accurate and reliable data. But what happens when this data is riddled with errors, inconsistencies, or duplicates? The consequences can range from poor decision-making to missed opportunities and tarnished customer relationships. This is where the data cleansing process becomes an indispensable element of a successful business strategy.
This blog delves into why businesses must prioritize data cleansing, its transformative benefits, and how to implement it effectively. If you’ve ever questioned the ROI of clean data, read on—this might just reshape your perspective.
The hidden costs of dirty data
Data is the backbone of modern business. But when it’s riddled with errors, it becomes a liability. Gartner estimates that poor data quality costs organizations an average of $12.9 million annually. Here’s how dirty data impacts businesses:
- Wasted resources: Employees spend more time fixing errors than innovating.
- Missed opportunities: Flawed data leads to missed sales or misguided campaigns.
- Damaged reputation: Sending incorrect information to customers erodes trust.
What is the data cleansing process?
The data cleansing process involves detecting, correcting, and removing inaccuracies, duplicates, and inconsistencies within datasets. It ensures data is accurate, consistent, and reliable—ready for decision-making and analytics.
Key steps in the process:
- Data profiling: Analyze datasets to uncover patterns and inconsistencies.
- Error detection: Identify duplicates, missing fields, or outliers.
- Correction or removal: Fix errors or eliminate irrelevant records.
- Standardization: Apply uniform formats and rules across datasets.
Why data cleansing matters
1. Empowering decision-making
Accurate data fuels effective strategies.
2. Enhancing customer relationships
Clean data helps businesses personalize customer interactions.
3. Regulatory compliance
Data protection laws like GDPR and CCPA demand data accuracy. Regular cleansing safeguards businesses against penalties while ensuring ethical data usage.
4. Boosting operational efficiency
With accurate datasets, teams can focus on innovation instead of troubleshooting errors.
Transformational outcomes of the data cleansing process
Best practices for effective data cleansing
- Automate repetitive tasks: Use modern data quality tools like Talend or OpenRefine to minimize manual effort.
- Set data governance rules: Define clear standards for data entry and maintenance.
- Regular audits: Schedule periodic checks to identify and resolve issues proactively.
- Integrate across systems: Synchronize cleansing efforts across all databases.
- Employee training: Equip teams with the skills to manage and maintain clean data effectively.
Read more: Data center migration checklist: Planning, execution, and post-migration essentials
Emerging trends in data cleansing
- AI-driven cleansing tools: Platforms like Informatica and Trifacta leverage AI to detect errors and inconsistencies more effectively.
- Proactive data quality monitoring: Modern solutions offer real-time alerts to maintain data integrity.
- Integration with advanced analytics: Clean data feeds predictive models, ensuring more accurate forecasts and insights.
- Data Democratization: Organizations increasingly empower non-technical users with tools to cleanse and analyze data without deep technical knowledge, fostering cross-departmental collaboration and efficiency.
- Predictive Data Maintenance: Leveraging machine learning, systems can now proactively predict and flag potential data quality issues before they arise, reducing errors.
Read more: Top innovations 2025: Redefining the world around us
Take the first step towards cleaner data
Clean data is more than an operational requirement—it’s a strategic asset. From better decision-making to enhanced customer satisfaction, the data cleansing process unlocks untapped potential in your business. Our data engineering services include data profiling, quality management, and data cleansing, which are crucial for maintaining accurate and reliable datasets. Further, our AI business solutions encompass data cleaning and preparation.
Ready to transform your data? Contact us for expert consultation and tailored solutions.